General Overview on LLM's
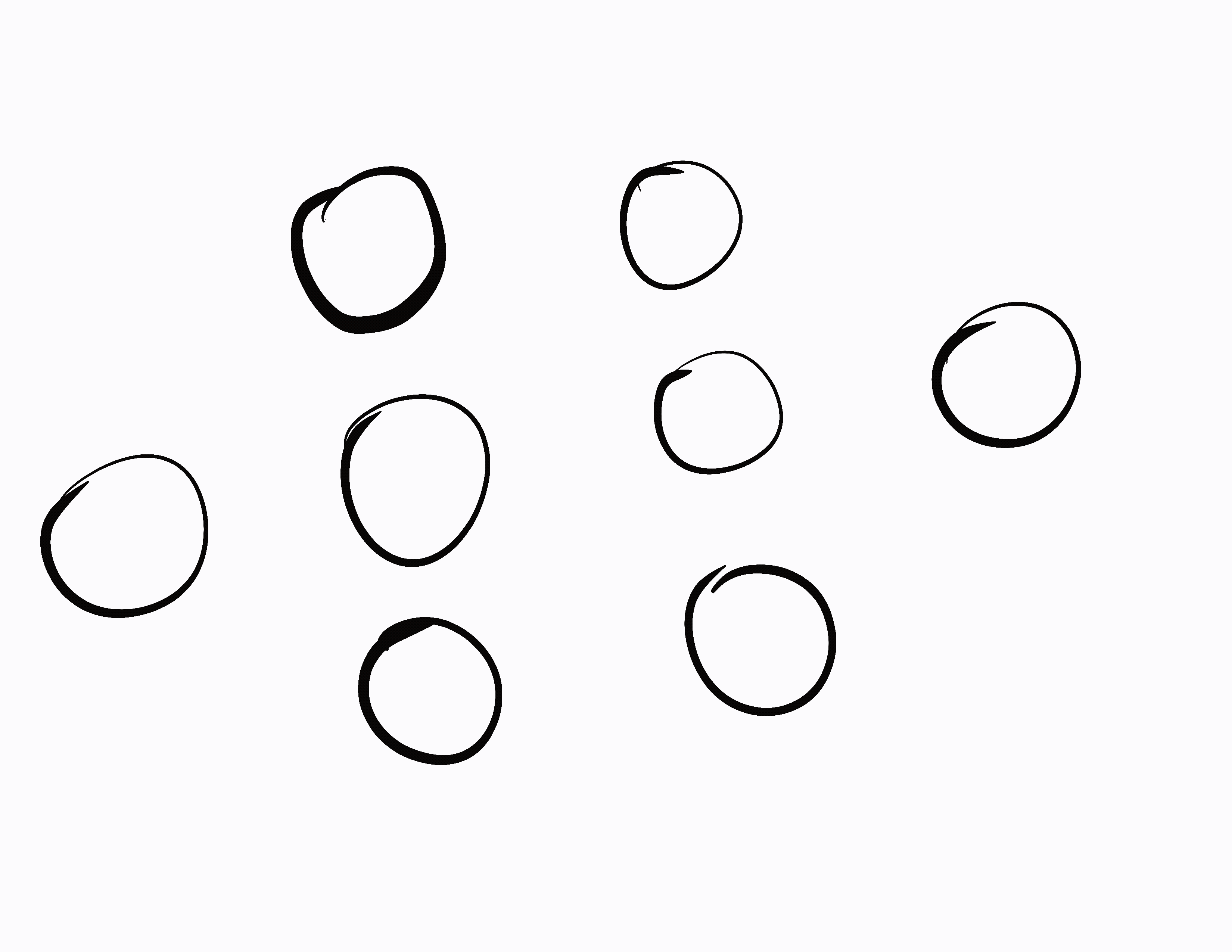
ML
This blog does not emphasize the idea of writing code to implement a Large Language Model (except for implementing the BERTopic algorithm at the end for beginners). Instead, it mainly focuses on providing a simple understanding of how these architectures work.
Before delving into these architectures and the math behind Large Language Models, we need to understand what a Neural Network is. Neurons are entities that compute raw values.
With a rough idea of what a neural network is, it’s time to focus on the math. How does a neural network work? General convolutional networks train the model to recognize images, for example, the numbers 1, 2, 3, 4, and 5. What do these networks calculate?
The computer or the model in our case does not actually understand what a word or an image is. We need to make it understand these concepts.
If you look at the basic layer of an image, it’s just a bunch of patterns with colored pixels. We can simplify by focusing on black and white pixels, assigning values of 1 for white and 0 for black. Let’s say you input an image with 28x28 pixels. How do you feed this image into data?
First, the neural network generates random weights and biases for every neuron that connects to the hidden layer. It then calculates the output at each iteration and checks the corresponding result. Based on the result, it calculates the loss at each layer and adjusts the weights and biases. This process is repeated at each layer in a backward manner, called backpropagation.
However, there’s a problem with this approach: the model stops learning after a certain point due to the vanishing gradient problem if we use the sigmoid function. That’s why we often use ReLU (Rectified Linear Unit) as the activation function.
See the video below to know why!
To be Continued.. This is an unfinished blog..
🎥Videos
Generated all the videos with manim python library